Annotation of Bacterial Genomes and Community Sequences: Genes, Operons, Promoters, Terminators, Protein Sub-Cellular Localization.
Softberry software for analysis of bacterial genomes:
- Automatic annotation pipeline Fgenesb
( see flowchart), (445 citation of using in matagenomic projects according to Google scholar),
which includes self-training genefinding parameters, prediction of CDS
and identification of closest protein homolog from COG, KEGG and NR
databases, mapping rRNA and tRNA genes, prediction of operons, promoters
and terminators. Suitable for both eu- and archebacterial genomes, and
for environmental samples of bacterial DNA sequences.
- Togenbank: A set of scripts to convert FgenesB output to GenBank
and Sequin formats, for visualization in popular viewers like Artemis
and for submitting annotated sequences to Genbank.
- Absplit:
A program that sorts bacterial sequences into eu- and archebacterial
subsets.
- BPROM:
Bacterial sigma70 promoter prediction program (831 citations according to Google scholar).
- FindTerm:
Finder of rho-independent terminators.
- ProtCompB:
Program for prediction of localization of proteins in bacterial cellular
compartments.
- Bacterial GenomeExplorer:
Interactive genome viewer with search capabilities, which lets user
to vizualise known and predicted genes, mRNAs, EST, promoters, terminators,
and other features.
- Several
programs for alignment and comparison of bacterial genomes.
- Visualization
of FgenesB annotation using CGView
- Human Microbiome gene prediction
- Softberry Microbiome Annotation Database
A set of bacterial genome annotation tools listed above
was successfully used in first published annotation of bacterial communities
(Community structure and metabolism through reconstruction of microbial
genomes from the environment, Nature 2004, 428, 37 - 43), and now
is widely used in dozens academic and industrial labs for sequence analysis
in bacteria.
Evaluations:
Comparative accuracy estimated on comprehensive tests: Mavromatis et al.
VOL.4 NO.6 | JUNE 2007 | NATURE METHODS:
The first, fgenesb pipeline was used to predict coding sequences both on the
assembled contigs and the unassembled reads. The second pipeline used a
combination of CRITICA and GLIMMER (called here CG pipeline). "Fgenesb
correctly identified 10-30% more reference genes on the contigs than the
CG pipeline in every data set (Fig. 2a). Both pipelines called 7-15% of
the genes inaccurately (Fig. 2a)"
"We also evaluated the accuracy of the gene calls on unassembled reads
(where low-abundance species are usually represented). Fgenesb correctly
identified ~70% and missed ~20% of reference genes on unassembled reads in all
data sets (Fig. 2b). The remaining 10% of reference genes were inaccurately
called and another ~8% were newly predicted. Notably, the contribution of
assembly to accurate gene prediction was not more than 20%, whereas its effect
on the missed and the inaccurately predicted genes was only slightly higher.
The CG pipeline exhibited poor results (7% accurately predicted, 85% missed
and 8% inaccurately predicted genes), and we did not use these data for the
following steps of the analysis".
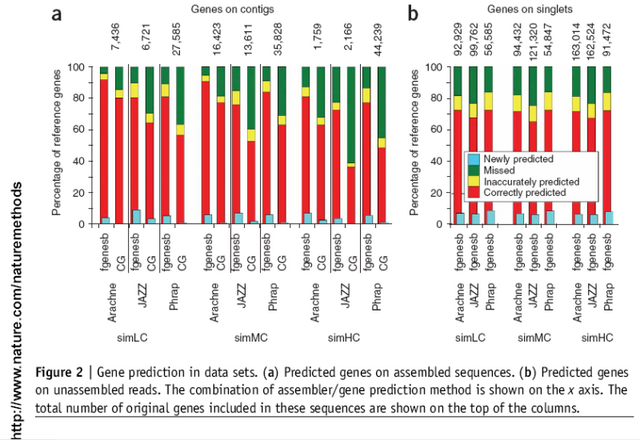
Prediction results on three sets of difficult to predict
bacterial genes for GeneMarkS and Glimmer (calculated in Nucleic Acids
Research, 2001, Vol. 29, No. 12, 2607-2618) and FgenesB (calculated by
Softberry):
|
123 set |
72 set |
51 set |
B.subtilis genome |
|
Sn (Sn_ov) |
Sn (Sn_ov) |
Sn (Sn_ov) |
Sn (Sn_ov) |
Glimmer |
57.0 (91.1) |
57.0 (88.2) |
51.0 (88.2) |
62.4 (98.1) |
GeneMarkS |
82.9 (91.9) |
88.9 (94.1) |
90.2 (94.1) |
83.2 (96.7) |
FgenesB |
89.3 (98.4) | 91.5 (98.6) | 92.0 (98.0) | 83.8 (98.7) |
Sn (Sensitivity), percentage of existing CDS predicted exactly
right. Sn_ov, percentage of CDS predicted exactly and with overlap. For
B.subtilis, we compared genes predicted by three programs with
all annotated genes in GenBank entry for that genome.
Application of Softberry programs for bacterial genomic and metagenomic sequence analysis in selected publications:
FgenesB:
Journal of Bacteriology (2008) 190, 4859-4864;
Journal of Bacteriology (2008) 190, 3646-3657;
Journal of Bacteriology (2008) 190, 3362-3373;
Journal of Bacteriology (2008) 190, 2777-2789;
Microbiology (2008) 154, 1422-1435;
Nature Methods (2007) 4, 495-500; PNAS (2007) 104 ,29, 11889-11894;
Nature Biotechnology (2007) 25, 447-453; PNAS (2007) 104 ,13, 5590-5595;
Nature Biotechnology (2006) 24, 1263-1269; PNAS (2006) 103, 34, 12897-12902;
Journal of Bacteriology (2006) 188, 14, 5228-5239; Microbiology (2006) 152, 1951-1968;
Molecular Microbiology (2006) 61, 4, 960-977; Nature (2006) 439, 847-850;
PLoS Biol (2006) 4,4 e95; Science (2005) 308, 554-557; Nature (2004) 428, 37-43.
BPROM:
Journal of Bacteriology (2008) 190, 4559-4567;
Journal of Bacteriology (2008) 190, 3877-3885;
Journal of Bacteriology (2008) 190, 2096-2105;
Microbiology (2008) 154, 1719-28;
Microbiology (2008) 154, 1372-83;
Infection and Immunity (2007) 75, 9, 4506-4513; Journal of Bacteriology (2007) 189, 2, 351-362;
Cellular Microbiology (2007) 9, 4, 1039-1049; Journal of Molecular Biology (2007) 368, 4, 966-981;
PNAS (2006) 103, 34, 12897-12902; Journal of Bacteriology (2006) 188, 14, 5089-5100;
Applied and Environmental Microbiology (2006) 72(4), 2539-2546; Molecular Microbiology (2006) 59, 2, 541-550;
Applied and Environmental Microbiology, (2006) 72, 1, 368-377; Journal of Bacteriology (2006) 188, 2, 789-793;
FEBS Journal (2005) 272, 24, 6324-6335; Infection and Immunity (2005) 73, 5, 2899-2909.
FindTerm:
Microbiology (2008) 154, 1422-1435;
Journal of Bacteriology (2008) 190, 3646-3657;
Microbiology (2007) 153, 2148-2158; Infection and Immunity (2007) 75, 9,4482-4489;
Enzyme and Microbial Technology (2007) 4, 5, 747-753; Journal of Bacteriology, (2006) 188, 1, 160-168;
Molecular Microbiology (2006) 59, 2, 541-550; Journal of Bacteriology (2006) 188, 1, 202-210;
Journal of Bacteriology (2006) 188, 1, 160-168.
|
|